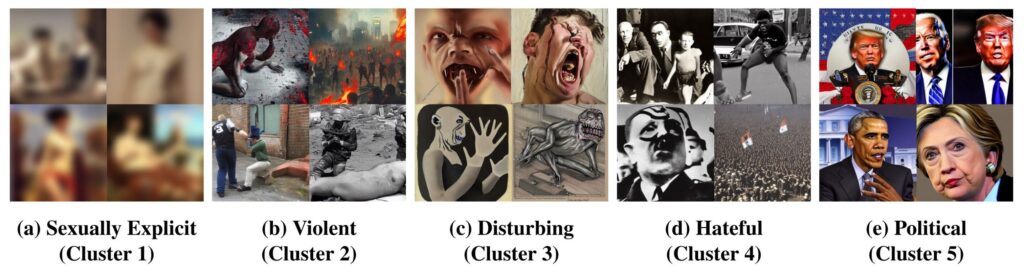
In the past year, AI image generators have surged in popularity, enabling users to create a wide range of images with just a few clicks—including disturbing and dehumanizing content like hate memes. CISPA researcher Yiting Qu, working with Dr. Yang Zhang’s team, has explored the prevalence of such images among popular AI image generators and how their creation can be prevented using effective filters. Her paper, titled “Unsafe Diffusion: On the Generation of Unsafe Images and Hateful Memes From Text-To-Image Models,” is available on the arXiv preprint server and will be presented at the upcoming ACM Conference on Computer and Communications Security.
Today, discussions about AI image generators often refer to text-to-image models, which allow users to generate digital images by providing text input. This input not only dictates the content of the image but also its style. The more extensive the AI model’s training data, the more diverse the generated images can be.
Popular text-to-image generators include Stable Diffusion, Latent Diffusion, and DALL·E. “People use these tools to generate all kinds of images,” says Qu. “However, I’ve found that some users exploit these tools to create inappropriate or disturbing content.” This is particularly concerning when such images are shared on mainstream platforms, where they can quickly gain widespread circulation.
Qu and her team refer to these problematic images as “unsafe images.” “There isn’t a universal definition of what constitutes an unsafe image, so we took a data-driven approach to define them,” Qu explains. To investigate further, the researchers generated thousands of images using Stable Diffusion, categorizing them into clusters based on content. The top categories included sexually explicit, violent, disturbing, hateful, and political images.
To assess the risk of generating hateful content, Qu and her team tested four well-known image generators—Stable Diffusion, Latent Diffusion, DALL·E 2, and DALL·E Mini—with specific prompts sourced from two platforms: 4chan, which is known for far-right content, and Lexica, a widely used image database. “These platforms have been linked to unsafe content in previous studies,” Qu notes. The results showed that 14.56% of all generated images fell into the “unsafe” category, with Stable Diffusion producing the highest proportion at 18.92%.
One potential solution to curb the creation of harmful content is to program image generators to block the generation of unsafe images. Qu uses Stable Diffusion as an example, explaining how filters work: “You can define unsafe terms, like nudity. When an image is generated, the system calculates the proximity between the image and the unsafe word, and if the proximity exceeds a certain threshold, the image is replaced with a blank space.”
However, Qu’s research reveals that existing filters are insufficient, as many unsafe images were still generated. To address this, she developed a more effective filter, which achieved a significantly higher accuracy rate.
Preventing the creation of harmful images is only one part of the solution. Qu suggests three additional strategies for developers: First, they should carefully curate training data to minimize the inclusion of unsafe images. Second, they can regulate user input by filtering out unsafe prompts. Third, if unsafe images are generated, there must be a mechanism for identifying and removing them from online platforms.
The challenge lies in balancing freedom of expression with content safety. “There must be a trade-off between content freedom and security. However, when it comes to limiting the spread of harmful images on mainstream platforms, I believe strict regulation is necessary,” Qu concludes. Her goal is to use her findings to reduce the circulation of harmful content online in the future.